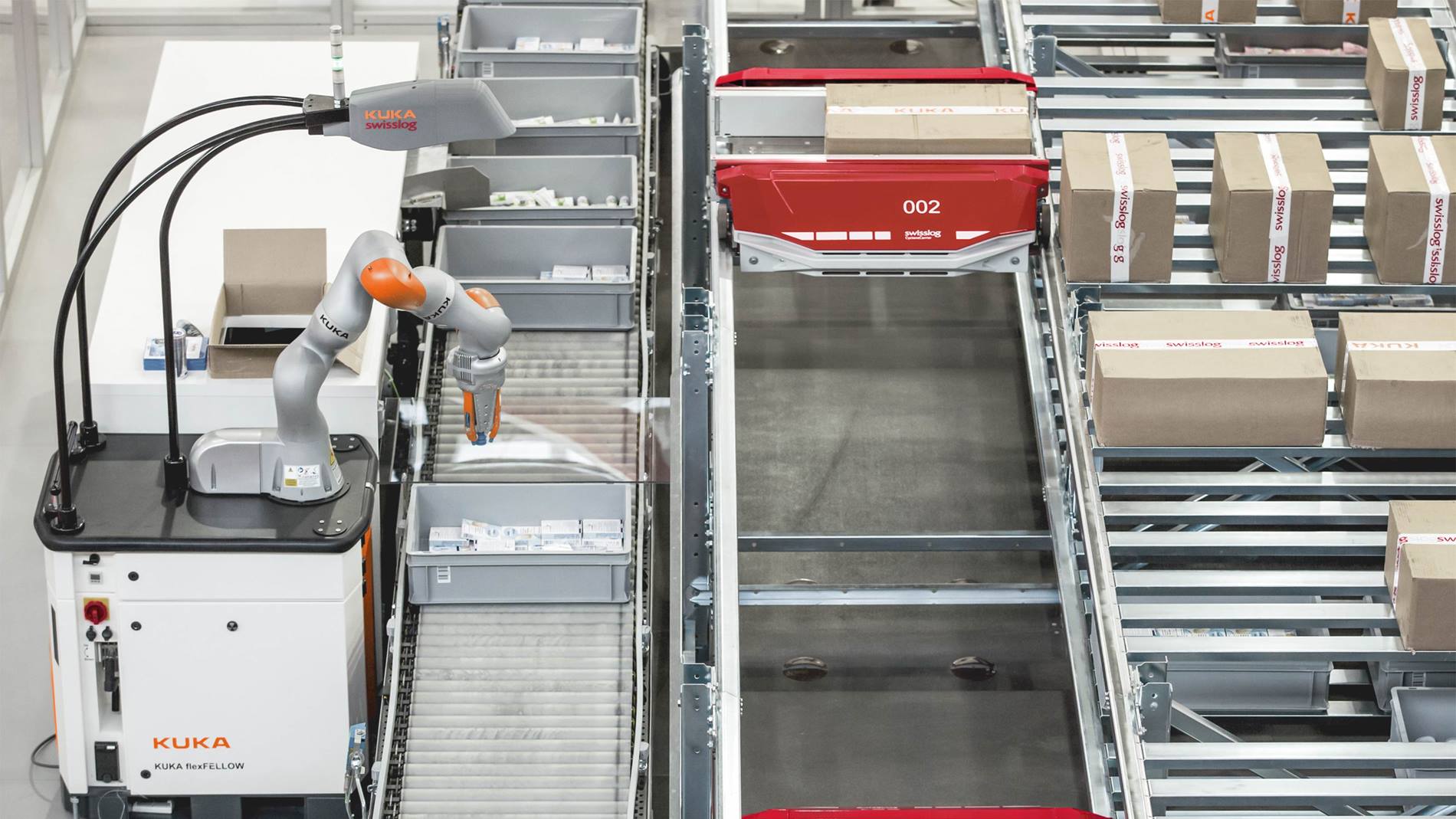
The Key to Robotic Picking is to Learn Like a Baby
January 4, 2018
Machine learning is key to the future of robotic item picking. But how will it overcome the three V’s of volume, velocity and veracity? The key may be in mimicking how babies learn.
In one of our previous posts, we outlined the challenges of robotic picking by highlighting the amazing capabilities of the human hand and its connection to the eyes, which makes it simple for us to reach into a bin and select a specific object from a group of random products.
This is not so easy for robotic pickers. They must break down what to us seems like one task, into multiple discrete tasks and decisions. We also shared some of the incredible progress that is taking place in the areas of vision and gripping technology that are allowing robots to close the gap with the human hand-eye combination.
But there is another piece to this puzzle: hand-eye coordination is made possible by the human brain. The brain is an excellent platform for doing complex tasks without the need for discreet programming. One of the key characteristics of the brain is its adaptability. Humans can learn from experience and improve their performance based on that experience. What is even more impressive is that humans can make decisions based on experience about how to proceed in situations we haven’t encountered before. Can robots be given this same ability?
That’s essentially what machine learning, a form of artificial intelligence, is all about. It is the ability of software embedded in devices such as robots, or operating in the cloud, to learn without being explicitly programmed. Machine learning is driving rapid advances in artificial intelligence and is already showing up in devices like our mobile phones, which can now predict what we are most likely to do next based on what we have done in the past.
Machine learning has a number of potential applications in the supply chain. Supply chain software, for example, is gaining the ability to track and predict disruptions by using machine learning and associative algorithms to correlate data between supply chain systems and external sources, such as social media, newsfeeds, and weather forecasts. It will also prove to be key in enabling robotic item pickers to learn to pick items faster and more accurately.
Yet, while there are a few innovators and early adopters, many in the warehousing industry remain skeptical. And there are goods reasons for their skepticism. The challenges to applying machine learning are parallel to the “three V’s” associated with Big Data applications: volume, variety, and veracity. Can this application of machine learning handle the huge amounts of data, can it correlate different types of data and can the data be trusted?
While these are valid concerns, we believe there is a path forward that will address them and it involves enabling robotic item pickers to learn the way human babies learn.
Humans are born with a grasping reflex. One of the small joys in life is putting your finger in a newborn’s palm and feeling their grip. But early in life, gripping is a reflex, not a voluntary action. At about four months, babies start to reach out and grasp items around them. As they do, they learn to recognize shapes. Before they learn the difference between red and blue, they learn the difference between, for example, a box and sphere and the best way to pick up and hold each. The more they grasp and hold the objects around them, the more adept they become at gripping and handling those objects.
This same approach can be applied to robotic item picking. Before robots attempt to learn the hundreds of thousands of SKUs in the typical retail warehouse, they need to learn the shapes of the various products represented by those SKUs and the best way to handle each. This is a necessary step forward because not only do those SKUs represent a huge volume of data, but they are constantly changing.
If you operate a retail warehouse, you know what I’m talking about. What you thought was a case of product all with the same SKU actually contains 10 SKUs, each with subtle differences. Or a product SKU hasn’t changed, but the product has, now offering 30% more in a special promotional pack. Or, the product is essentially the same, but is now part of a sweepstakes and so has a new SKU. The number of variations is incredible.
So, instead of expecting robotic item pickers to adapt to each change, which severely limits their ability to support the picking speeds that modern retail warehouses require, machine learning can be more effectively employed by enabling robots to learn to efficiently handle the variety of shapes represented by the typical retail product mix.
As a division of KUKA, one of the world’s largest robotics companies, we are bullish on the future of robotics in material handling. As with our other offerings, our goal is to provide e-commerce and retail warehouses with solutions that can adapt to inevitable changes in products and markets. Using machine learning to optimize how robots handle various shapes is critical to that effort and will enable robotic item pickers to bring new levels of productivity and flexibility to the retail/e-commerce warehouse sooner than many in the industry expect.